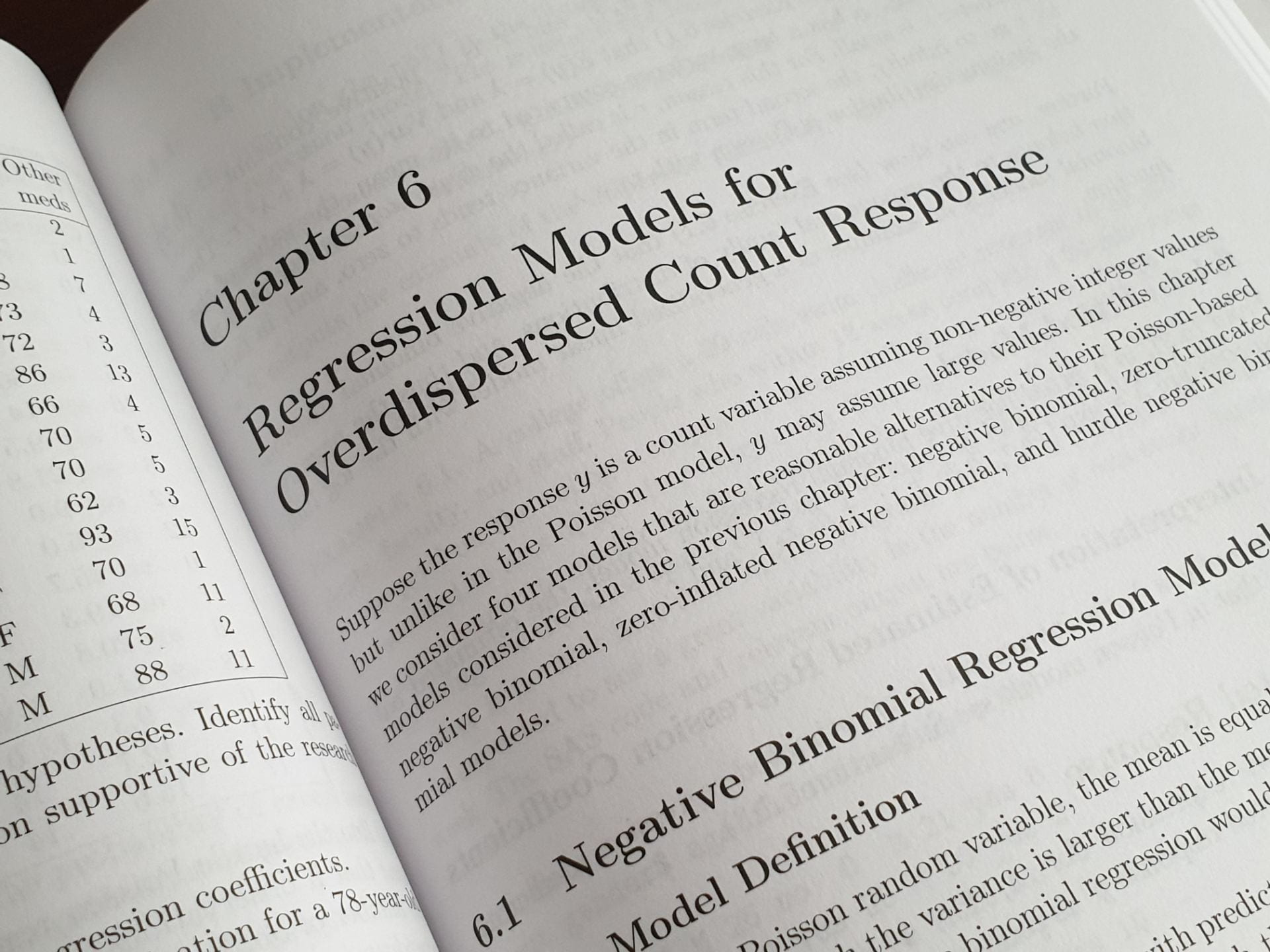
Tuesday October 22, 2020 – 12:15-13:45pm – online
Predictive regressions: a machine learning perspective
Dr. Guillaume Coqueret, emlyon business school
Abstract: We characterize the quadratic loss that is expected when forecasting an autocorrelated process from its linear relationship with another autocorrelated process. When the predictions are based on sample estimates, we link the accuracy to three key quantities: the persistence of the underlying series, the forecasting horizon, and the sample size. We find that high autocorrelation in the dependent variable is required to reach reasonably low levels of losses. In this case, we identify two configurations which generate positive out-of-sample R^2: short term forecasting with small samples and long horizon predictions with very deep samples. They correspond to two canonical variables in financial economics: the short term volatility and the long term equity premium. We confirm our results via an empirical study on the S\&P 500 with a series of 15 popular predictors in the literature.
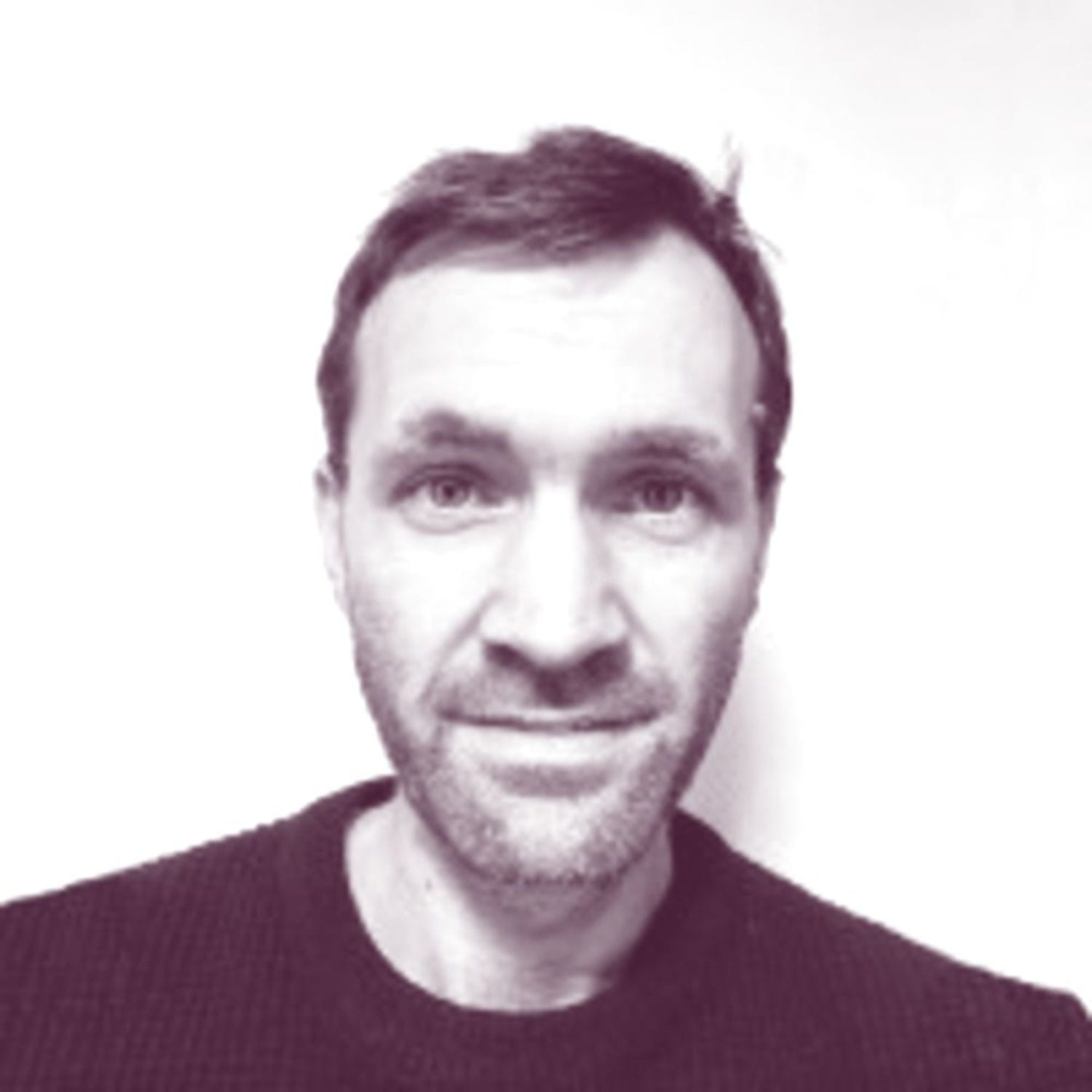
Guillaume is an associate professor of Finance & Data Science at emlyon business school. His research interests revolve around applications of numerical methods in various fields (financial economics mostly). Some of his recent work focuses on supervised learning and on sustainable equity investing.