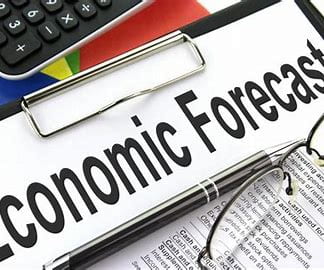
emlyon faculty: Dr. Guillaume Coqueret
in collaborations with: Mr. Romain Deguest (independent)
We characterize the quadratic loss that occurs when forecasting an autocorrelated process from its relationship with another autocorrelated process. When the predictions are based on sample coefficients, we link the accuracy to three key quantities: the persistence of the underlying series, the forecasting horizon, and the sample size. Their impacts have mitigating effects, which creates a memory tradeoff. We surprisingly illustrate that choosing predictors with high autocorrelation often leads to lower performance, especially when the the autocorrelation of the predicted variable is high. We confirm our results with an empirical study on the S&P 500 with a series of 15 popular predictors in the literature.