A big data-driven framework for solving class imbalance problem without cost tuning
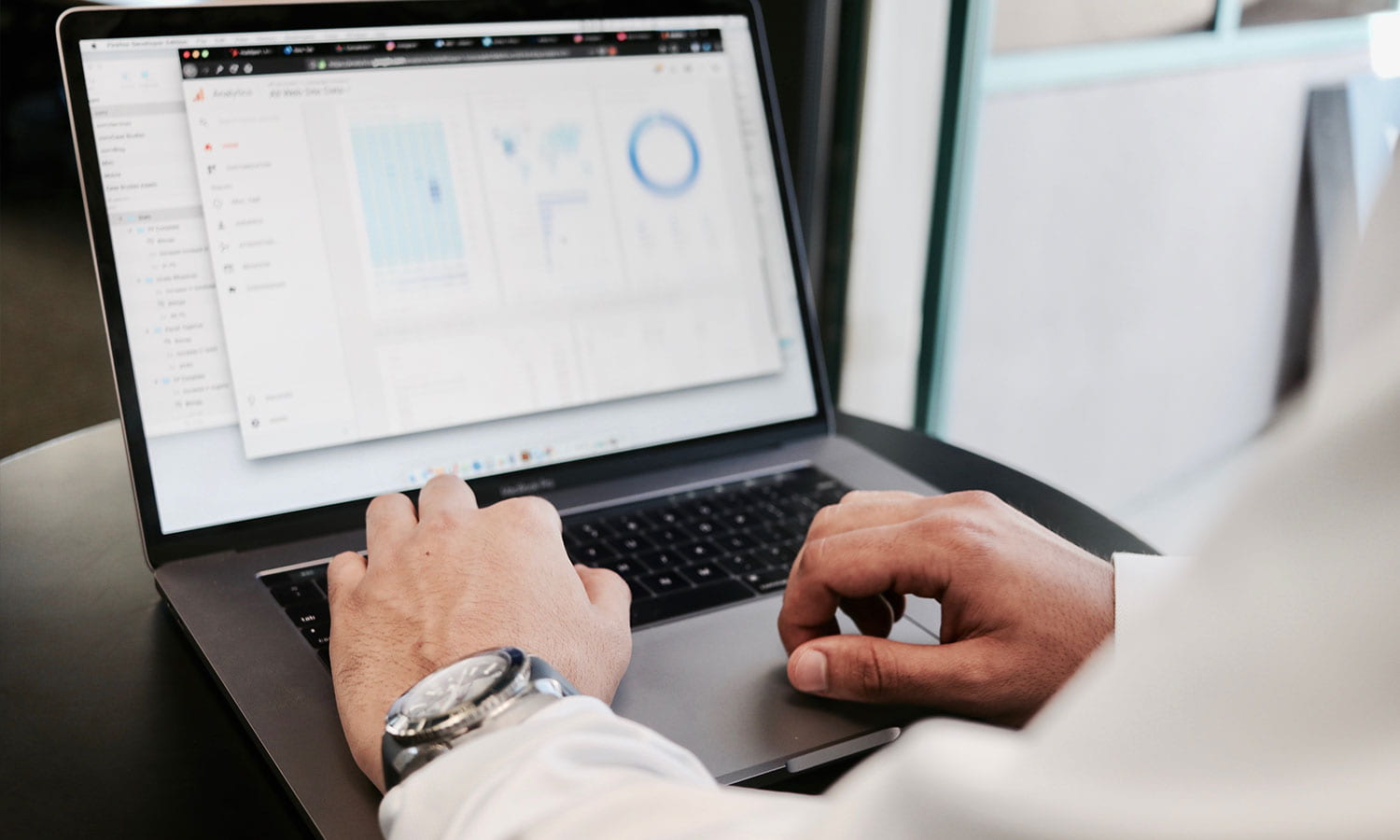
Ajay Kumar (emlyon business school), Ravi Shankar (IIT Delhi)
Learning from class-imbalanced data is one of the most challenging problems in supervised machine learning, so many different strategies are designed to tackle balanced sample distribution. Many algorithms have been proposed for solving this task, but most are complex and tend to generate unnecessary noise. In this work, we first investigate the effects of several over-and under-sampling, cost-sensitive learning and boosting techniques on the problem of learning from imbalanced behavior data and then we develop an algorithm, which avoids the generation of noise and effectively overcomes imbalances between and within classes. We applied our proposed algorithm on 20 publicly available benchmark imbalanced data-sets and empirical results of extensive experiments show that training data over-sampled with the proposed algorithm improves classification results.